The Role of AI in Scientific Research: How Machines are Accelerating Discoveries
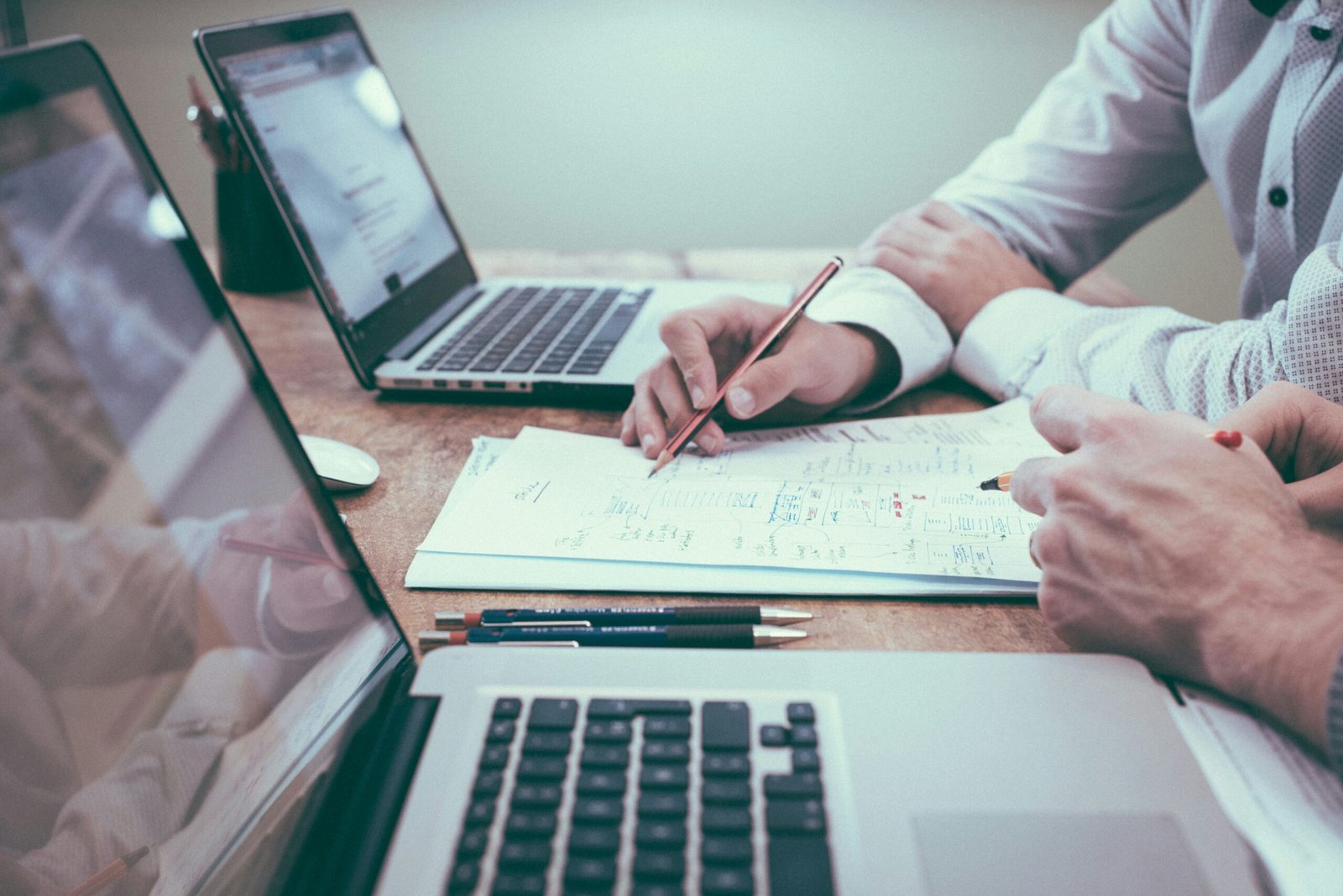
Introduction to AI in Scientific Research
The advent of artificial intelligence (AI) marks a pivotal moment in the evolution of scientific research, as it introduces new methodologies and perspectives that significantly enhance traditional approaches. AI encompasses a range of technologies, including machine learning, natural language processing, and data mining, all designed to analyze complex datasets, identify patterns, and facilitate informed decision-making. As researchers increasingly turn to these advanced tools, the relevance of AI within the scientific community continues to grow.
One of the most profound impacts of AI in scientific research is its ability to manage and analyze vast amounts of data—something that is becoming increasingly vital in today’s data-driven environment. In fields such as genomics, medical research, and environmental science, AI systems can swiftly process and interpret data, enabling researchers to derive insights that would take significantly longer using traditional methods. Through the automation of repetitive tasks and sophisticated predictive modeling, AI not only expedites research workflows but also enhances accuracy and consistency.
Moreover, AI technologies also assist in hypothesis generation and experimental design. By leveraging existing knowledge and previous research, AI can suggest new avenues for inquiries or better methods to test hypotheses. This synergy between human researchers and machine intelligence fosters innovative problem-solving, ultimately propelling the pace of scientific discovery to unprecedented levels. The promise of AI extends across various disciplines, democratizing access to cutting-edge tools that were once limited to elite research institutions.
As we explore the role of AI in scientific endeavors, it is critical to acknowledge its transformative potential and the opportunities it presents for accelerated discoveries. The integration of AI into research not only signifies a shift in how science is conducted but also hints at a future where the boundaries of knowledge are expanded, driven by the capabilities of intelligent machines.
AI Technologies: Types and Applications
Artificial Intelligence (AI) technologies have become increasingly vital in scientific research, enabling researchers to address complex problems and accelerate discoveries. Among the diverse spectrum of AI, three prominent categories stand out: machine learning, natural language processing, and robotics. Each of these technologies offers unique advantages and applications, enhancing the efficiency and effectiveness of research processes.
Machine learning, a subset of AI, allows systems to learn from data and improve iteratively without explicit programming. This capability is pivotal in data analysis, where massive datasets can be processed more rapidly and accurately than traditional methods. By employing machine learning algorithms, researchers can uncover patterns and make predictions based on historical data, significantly informing decision-making processes in fields such as genomics and climate modeling.
Natural language processing (NLP) is another essential AI technology that focuses on the interaction between computers and human language. In scientific research, NLP can be used to analyze vast amounts of scientific literature, helping researchers identify relevant information, summarize findings, and extract key insights. Tools powered by NLP have shown remarkable success in systematically reviewing articles and extracting relevant data, saving time and resources for researchers while increasing the thoroughness of literature reviews.
Robotics, the third key area, plays a transformative role in automating experiments and collecting data. Through the use of robotic systems, researchers can conduct experiments with greater precision and repeatability. These systems can carry out complex tasks such as high-throughput screening in drug discovery or automated sample preparation in laboratory settings. Such innovations not only increase productivity but also facilitate experimentation that may have been impractical or impossible to conduct manually.
Overall, the integration of AI technologies—machine learning, natural language processing, and robotics—into scientific research marks a significant advancement in methodologies, enabling researchers to operate at an unprecedented scale and speed while driving innovation across various disciplines.
Data Analysis Revolutionized by AI
The advent of artificial intelligence (AI) has significantly transformed the landscape of scientific research, particularly in the realm of data analysis. In contemporary research, the volume of data generated is unprecedented, presenting both opportunities and challenges for scientists. Traditional methods of data handling often struggle to keep pace with the sheer scale and complexity of information now available. AI algorithms, however, have emerged as powerful tools that can process vast datasets efficiently and effectively, thus revolutionizing how researchers approach their work.
AI techniques, such as machine learning and deep learning, enable the analysis of complex datasets that would traditionally take human researchers an extended period to interpret. For instance, machine learning algorithms can identify patterns within scientific data that might be imperceptible to the naked eye, allowing for faster insights into findings. This capability is particularly important in domains such as genomics and environmental science, where the intertwining variables can lead to nuanced understanding and significant discoveries. Notably, AI-driven tools like TensorFlow and PyTorch are empowering researchers to create models that enhance predictive accuracy, which is critical in hypothesis testing and validating research outcomes.
Moreover, AI’s capacity to streamline data processing contributes to improved speed and accuracy in research. By employing algorithms designed to handle specific tasks, researchers can save valuable time, enabling them to focus on interpretation and application of their findings. For example, AI applications can automate repetitive tasks such as data cleaning, which not only reduces human error but also enhances the consistency of data analysis. As a result, scientists can leverage AI to delve deeper into their studies, driving innovation and paving the way for accelerated scientific discoveries that benefit society as a whole.
Accelerating Drug Discovery with AI
The landscape of drug discovery has been undergoing a significant transformation due to the integration of artificial intelligence (AI). Traditionally, this process has been characterized by long timelines and substantial financial investments, often taking over a decade and costing billions of dollars to bring a new drug to market. AI is now poised to expedite this process, leveraging its capabilities to identify potential drug candidates more efficiently than ever before.
One of the primary applications of AI in drug discovery involves the identification of new drug candidates. Machine learning algorithms can analyze vast datasets from chemical libraries and biological samples to recognize patterns that may indicate promising compounds. This automation reduces the time researchers spend on routine tasks, allowing them to focus on more complex problems. Furthermore, AI can optimize molecular structures, improving the efficacy and safety profiles of potential drugs by predicting how they will interact with biological targets.
Predicting biological responses is another crucial area where AI demonstrates its potential. Machine learning models can forecast how different compounds will behave in the body, thereby facilitating the selection of candidates that are more likely to succeed in clinical trials. This predictive capability not only streamlines the research process but also minimizes the risk and cost associated with late-stage failures.
Several case studies highlight the effectiveness of AI in pharmaceutical research. For instance, a prominent AI platform was able to identify a novel antibiotic in a matter of days, a task that would typically require months or years of laboratory work. Similarly, another pharmaceutical company deployed machine learning to repurpose existing drugs for new therapeutic uses, significantly cutting down on the time required for development.
As AI continues to evolve, its role in the drug discovery process is likely to expand further, reinforcing the promise of faster, cheaper, and more successful innovations in medicine.
Enhancing Predictive Models in Scientific Research
Artificial intelligence (AI) has emerged as a transformative force in the realm of scientific research, particularly in the development of advanced predictive models. These models serve as essential tools that assist researchers in accurately forecasting complex phenomena across various domains, including climate science, epidemiology, and ecology. By leveraging sophisticated machine learning algorithms, scientists have been able to enhance the precision and reliability of their predictions, leading to more informed decision-making processes.
In climate science, for instance, researchers utilize AI-driven predictive models to analyze vast datasets related to weather patterns, atmospheric conditions, and greenhouse gas emissions. This enables them to make better forecasts regarding climate change impacts, such as extreme weather events and shifts in ecosystems. Enhanced predictive capabilities foster a deeper understanding of climate dynamics, allowing policymakers to formulate effective mitigation strategies more swiftly.
Similarly, in the field of epidemiology, AI plays a pivotal role in tracking disease outbreaks and predicting their spread. Machine learning algorithms can integrate information from numerous sources—such as healthcare data, social media, and geographic information systems—to develop models that predict the trajectory of infectious diseases. This level of enhanced predictive accuracy is critical for public health officials who must devise timely interventions to contain outbreaks and allocate resources efficiently.
In ecology, AI contributes to predictive models that monitor biodiversity and assess ecosystem health. By examining patterns in species populations and environmental variables, researchers can forecast potential declines in biodiversity or shifts in species distributions. This information is vital for conservation efforts and the sustainable management of natural resources.
Through these applications, AI not only refines the predictive capabilities of scientific research but also significantly improves the decision-making capabilities of researchers and policymakers across various fields. As technology continues to evolve, the potential for AI to further impact predictive modeling and enhance scientific inquiry remains promising.
Automation and Robotics in Research Laboratories
The integration of artificial intelligence (AI) and robotics within laboratory environments has revolutionized the landscape of scientific research. Automation has emerged as a cornerstone of modern laboratories, enabling researchers to conduct high-throughput experiments with unprecedented efficiency. By employing robotic systems for repetitive tasks, scientists can minimize human error, significantly mitigate time constraints, and enhance overall productivity in their research endeavors.
Robotic systems are particularly beneficial in fields such as chemistry and biology, where precision and accuracy are paramount. In chemistry labs, automated instruments can prepare and analyze compounds much faster than human researchers. For example, robotic arms can handle multiple samples simultaneously, allowing for the execution of experiments that would traditionally take days or even weeks. This not only accelerates the discovery process but also leads to improved data quality, as the likelihood of human-induced errors diminishes. Furthermore, the ability of these systems to consistently repeat experiments ensures that results are reproducible, a fundamental aspect of scientific inquiry.
In biological research, automation plays a critical role in genomics and drug discovery. High-throughput screening (HTS) methods executed by robotic platforms enable researchers to test thousands of compounds against biological targets in a fraction of the time required for manual processes. This rapid testing capability facilitates the identification of potential therapeutic agents and accelerates the development of new treatments. The implementation of AI algorithms can further enhance these robotic systems by predicting outcomes based on historical data, thereby optimizing experimental designs and resource allocation.
As laboratories continue to embrace the advancements in automation and robotics, the impact on scientific research will undoubtedly be profound. Through the adoption of these technologies, researchers can focus on innovative thinking and hypothesis generation rather than being bogged down by routine tasks, paving the way for groundbreaking discoveries.
AI Ethical Considerations and Challenges
The integration of artificial intelligence (AI) into scientific research introduces a multitude of ethical considerations and challenges that must be addressed to ensure responsible use. One of the foremost concerns lies in data privacy. As AI systems often rely on large datasets, the potential for personal data misuse becomes significant, especially in sensitive fields such as healthcare and genomics. Researchers must navigate the intricate balance between utilizing comprehensive data for advancing scientific knowledge and protecting the privacy of individuals whose data is being analyzed. Robust data governance frameworks are essential to mitigate such risks, emphasizing informed consent and secure data handling practices.
Another critical challenge pertains to biases in AI algorithms. The datasets used to train AI models can reflect historical biases, leading to skewed outcomes that may perpetuate inequalities. In scientific research, these biases can adversely affect the validity of findings and the applicability of results across diverse populations. It is therefore imperative for researchers to actively scrutinize and curate their datasets, ensuring diversity and representation. Implementing techniques such as algorithm auditing and bias detection can significantly enhance the fairness and accuracy of AI-driven research outcomes.
The transparency of AI decision-making processes is also a significant ethical concern. Many AI systems operate as black boxes, where the inner workings and rationale behind decisions remain obscure. In the realm of scientific research, where reproducibility and accountability are paramount, such opacity can undermine trust. Establishing clear guidelines for AI transparency, such as requiring explainable AI frameworks, allows researchers to better understand and justify AI-generated insights. Furthermore, fostering multidisciplinary collaborations, including ethicists and sociologists, can lead to the formulation of ethical guidelines that govern AI usage in scientific environments.
Collaboration Between AI and Human Researchers
The integration of artificial intelligence (AI) into scientific research has transformed the landscape of discovery, showcasing an unprecedented collaboration between human researchers and machines. Rather than serving as a replacement for the human intellect, AI is designed to augment and enhance researchers’ capabilities. This partnership enables a fusion of human intuition with the analytical power of AI, paving the way for groundbreaking innovations across various scientific disciplines.
One prominent example of this collaboration can be observed in the field of drug discovery. Researchers at major pharmaceutical companies have begun employing AI algorithms to analyze vast datasets, identifying potential drug candidates much faster than traditional methods. For instance, Insilico Medicine used AI to generate a novel molecule for a particular disease, effectively cutting down the time required for drug discovery from years to mere months. Here, human researchers guided the model with specific parameters, demonstrating how AI acts as a tool for accelerating their efforts, rather than replacing their experience.
Moreover, in climate science, AI models are instrumental in processing enormous datasets from satellites, providing insights into climate patterns and environmental changes. Scientists leverage these insights to inform policy decisions and develop mitigation strategies against climate change. This collaborative effort showcases the strength of interdisciplinary teamwork, where human researchers apply their contextual knowledge while AI analyzes complex variables, optimizing results in real time.
The synergistic relationship between AI and human researchers marks a pivotal shift in scientific inquiry. By harnessing the strengths of both human intellect and AI’s computational capabilities, they can tackle increasingly complex challenges. This collaborative framework not only accelerates discoveries but also ensures that human oversight and ethical considerations remain at the forefront of scientific advancements.
Future Directions: The Next Frontier of AI in Research
The integration of artificial intelligence (AI) into scientific research continues to evolve, presenting promising prospects for the future. As researchers increasingly rely on AI algorithms to analyze massive datasets, several emerging trends are indicative of the next wave of advancements in this field. One notable trend is the development of explainable AI (XAI), which aims to make AI decision-making processes more transparent and interpretable. This is particularly important in scientific contexts, where understanding the rationale behind algorithmic outputs can enhance trust in AI-generated insights.
Another significant area of focus is the application of machine learning techniques in the realm of personalized medicine. As data collection becomes more sophisticated, AI systems can analyze genomic information to identify tailored treatment plans for individual patients, thereby optimizing health outcomes. This personalized approach is expected to revolutionize not only healthcare but also fields such as pharmacology, where AI can help in early drug discovery by predicting how different compounds will interact with biological systems.
Moreover, the collaboration between AI and researchers is anticipated to expand across interdisciplinary domains. For example, AI’s capabilities in simulation and modeling can provide researchers in materials science and environmental studies with invaluable tools to predict the properties of new materials or assess ecological impacts of climate change. Such interdisciplinary collaborations promise to spur innovative solutions to some of the most pressing challenges faced by modern society.
Expert opinions highlight the importance of ethical considerations as AI technologies become pervasive in scientific research. Ensuring responsible AI use will require ongoing dialogue among scientists, ethicists, and policymakers to navigate the implications related to data privacy, bias, and accountability. As advancements unfold, it is crucial to establish frameworks that guide AI development while prioritizing integrity and human oversight in scientific endeavors.