The Role of Data Analytics in Modern Sports: From Recruitment to Performance
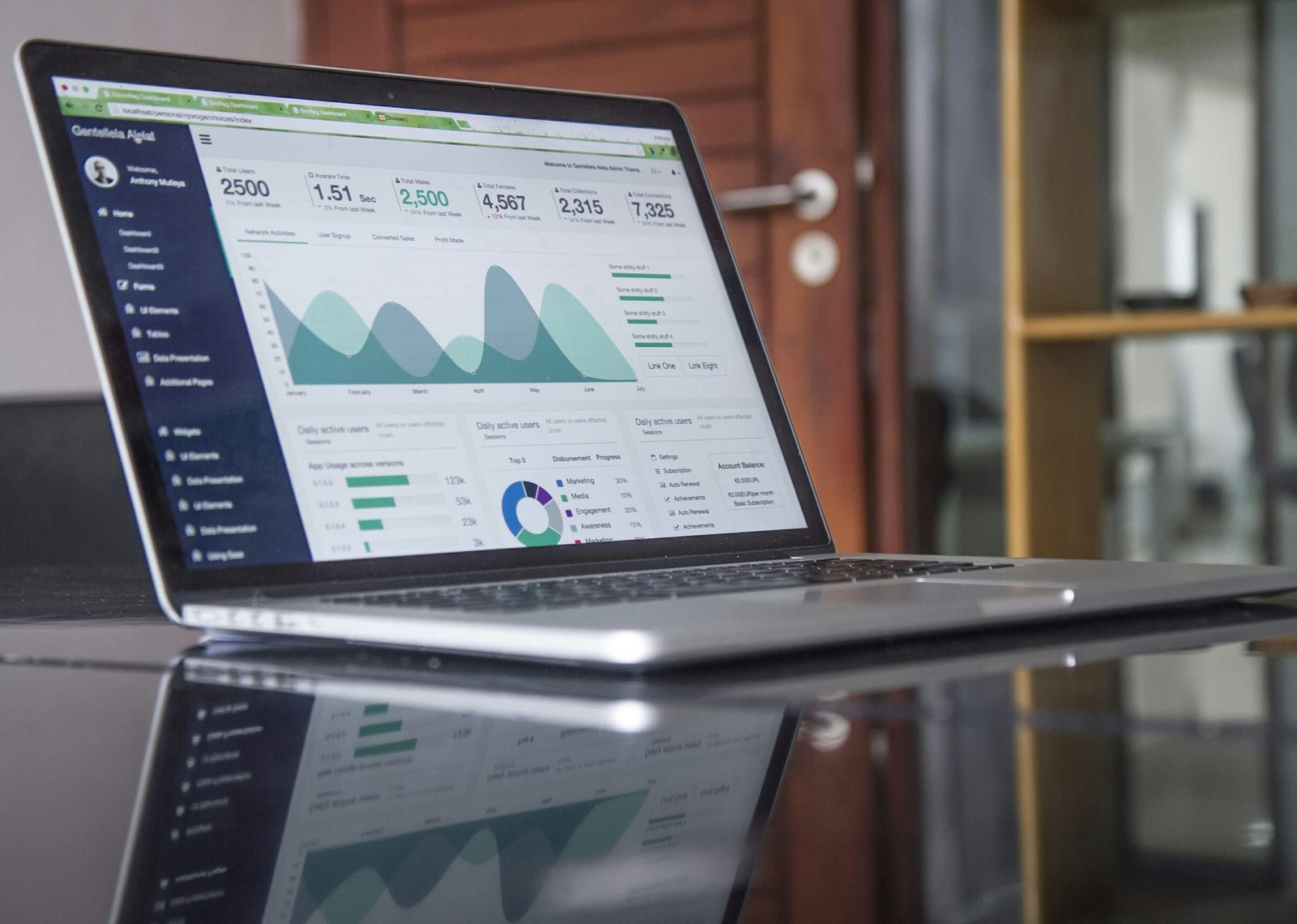
Introduction to Data Analytics in Sports
In recent years, the landscape of the sports industry has undergone a significant transformation, largely driven by the advancements in data analytics. This process involves the systematic collection and analysis of data to support decision-making within various sectors of sports, including player recruitment, training, game strategy, and injury prevention. The growing importance of data analytics in sports can be attributed to its ability to provide valuable insights which were previously unattainable through traditional methods. Teams and athletes now rely on data-driven strategies to enhance their performance and maintain competitive advantage.
At its core, data analytics in sports integrates a blend of technology and statistical methodologies to interpret player performance metrics and team dynamics. Key terms such as big data, machine learning, and predictive analytics are frequently encountered in this domain. Big data encompasses vast amounts of information generated from various sources, including player tracking systems, video analysis, and wearable technology. Machine learning, on the other hand, utilizes algorithms to analyze this data and identify patterns that can inform coaching strategies and player development.
Furthermore, predictive analytics plays a paramount role in foreseeing outcomes, allowing teams to make more informed choices regarding recruitment and game strategies. This data-driven approach not only holds the potential to improve athletic performance but also revolutionizes how teams analyze opponents and tailor their game plans accordingly. As sports organizations increasingly recognize the value of data analytics, the industry is set on a trajectory of continuous innovation and evolution, fundamentally changing the way sports are played and understood.
Historical Context: Evolution of Data in Sports
The integration of data analytics into sports has a rich historical context that dates back centuries. Traditionally, sports relied on basic statistics to assess player performance and team dynamics. For instance, in baseball, rudimentary stats such as batting averages and home runs were recorded as early as the 19th century. However, this initial phase represented only the tip of the iceberg in the evolution of sports analytics.
As the 20th century unfolded, the need for more sophisticated methods became apparent. The introduction of computer technology in the 1960s marked a significant turning point. Organizations began to utilize computers for data processing, allowing for a more systematic collection and analysis of performance metrics. This enabled teams to leverage statistical data, leading to enhanced decision-making processes regarding player recruitment and strategy formulation.
The 1980s and 1990s saw the emergence of advanced metrics, exemplified by the progressive adoption of the SABRmetrics movement in baseball, which introduced intricate statistical formulas to evaluate performance beyond traditional stats. This shift signaled a broader recognition of the importance of data in sports. Basketball also began leveraging data analytics with the development of unique performance metrics to assess player contributions more accurately.
With the advent of the Internet in the late 1990s and early 2000s, the scope of data collection dramatically expanded. Data became more accessible, leading to the concept of “Big Data,” where vast amounts of information could be processed and analyzed in real-time. This period laid the foundation for contemporary models of sports analytics, incorporating machine learning and complex algorithms capable of predicting outcomes, optimizing player performance, and informing coaching strategies.
The evolution of data analytics in sports thus reflects a progressive journey from simple statistics to intricate data models, revolutionizing how teams operate and compete at various levels. Through this historical lens, it is evident that data-driven decision-making has become an integral component of modern sports.
Recruitment and Scouting: Data-Driven Talent Identification
In recent years, data analytics has emerged as a powerful tool in the recruitment and scouting processes of professional sports teams. Coaches and scouts are increasingly relying on vast amounts of data to enhance their talent identification strategies. By analyzing player performance through an array of statistics, teams can gain insights into potential signings that would meet their competitive requirements.
Data analytics encompasses various methods, including the review of player statistics, detailed scouting reports, and advanced performance metrics. Traditional scouting methods are being augmented with data-driven strategies that provide a more comprehensive view of a player’s abilities. For instance, metrics such as player efficiency ratings, expected goals, and shot accuracy allow evaluators to make informed decisions based on quantifiable evidence. This shift allows teams to minimize reliance on subjective assessments and personal biases that may cloud judgment.
Several professional teams have demonstrated the success of integrating data analytics into their recruitment processes. For example, the baseball team Oakland Athletics famously utilized sabermetrics to build a competitive roster with limited financial resources. Their focus on overlooked talents who excelled in critical metrics led to a successful season, highlighting the effectiveness of data-driven recruitment strategies. Similarly, in soccer, clubs like FC Barcelona have employed data analytics through their La Masia academy to identify young prospects who possess distinctive attributes that fit the team’s playing style.
As technology continues to evolve, the breadth of data available is expected to expand even further. This continuous development will allow sports organizations to refine their recruitment processes even more. Ultimately, the integration of data analytics not only enhances the scouting capabilities but also plays an essential role in building competitive teams that have a better chance of success on the field.
Performance Analysis: Enhancing Athlete Efficiency
In the realm of modern sports, data analytics plays a crucial role in assessing and enhancing athlete performance. By leveraging advanced technologies such as wearables and game analytics software, coaches and athletes are equipped with the tools necessary to analyze performance data in real time. These technologies gather a wealth of information, including physiological metrics, movement patterns, and tactical decisions made during training and competitions.
Wearables, such as heart rate monitors, GPS trackers, and motion sensors, provide invaluable insights regarding an athlete’s physical conditions and biomechanical movements. These devices enable practitioners to monitor vital statistics, such as heart rates, distances covered, and acceleration patterns, allowing them to track an athlete’s workload and recovery status. This immediate feedback allows for timely adjustments to training regimens, tailoring them to optimize performance while minimizing the risk of injury.
Game analytics software further elevates the analysis process by providing detailed breakdowns of in-game performances. By using video analysis, coaches can dissect plays, evaluate decision-making, and identify both strengths and weaknesses in athlete performance. Automated tagging of key events during gameplay allows for swift retrieval of moments that need review, facilitating focused discussions between athletes and coaches. This collaborative analysis process helps in devising strategies aimed at improving specific aspects of performance.
The integration of these analytics tools generates actionable insights that can translate into enhanced training protocols. Coaches can identify trends and patterns in performance data, ensuring that training programs are adapted to meet individual athlete needs. By focusing on specific metrics that correlate with success in their respective sports, athletes can fine-tune their strategies and techniques, ultimately enhancing their efficiency on the field or court. This data-driven approach fosters a culture of continuous improvement, crucial for both individual athletes and teams striving for excellence.
Injury Prevention and Management: Leveraging Data for Health
In the realm of sports, injury prevention and management have become focal points for ensuring athlete longevity and optimal performance. The integration of data analytics plays a critical role in this domain by providing insights that help coaches, medical teams, and athletes themselves understand injury risks and enhance recovery protocols. Through the utilization of predictive analytics, organizations can now identify potential injury risks before they escalate into significant issues. By collecting and analyzing data on an athlete’s training loads, biomechanical patterns, and even nutrition, teams can tailor individual training regimens that minimize the likelihood of injury occurrence.
One prominent application of data analytics in injury management is the monitoring of athletes’ health metrics over time. By employing wearable technology, such as heart rate monitors and GPS devices, teams gather real-time data that provides a comprehensive view of an athlete’s physiological state. This data allows medical personnel to flag abnormal patterns that may signal fatigue, overtraining, or impending injury. Consequently, timely interventions can be made to adjust training loads or implement recovery strategies, thus safeguarding athlete health.
Examples of successful injury management programs abound in modern sports. For instance, the NBA utilizes advanced analytics to study player movements and identify stress factors that contribute to injuries. Programs incorporating machine learning algorithms examine vast datasets to predict the likelihood of injuries based on player behavior and context. In soccer, clubs like Manchester City have established robust health monitoring systems, which have recorded substantial reductions in injury rates after implementing data-driven training adjustments.
Overall, the adoption of data analytics in injury prevention and management is redefining how sports organizations approach athlete health. By proactively leveraging data insights, teams not only enhance their injury management strategies but also foster a culture of long-term athlete wellness, ultimately leading to improved performance outcomes.
Fan Engagement and Broadcasting: The Data-Driven Experience
In the contemporary landscape of sports, the integration of data analytics extends beyond the realms of team performance and athlete recruitment, significantly influencing fan engagement and the broadcasting experience. As organizations seek to deepen their connection with supporters, the strategic use of analytics becomes paramount. Insights derived from extensive data collection enable teams to understand fan preferences and behaviors, thereby facilitating a more personalized content delivery system. This customization can manifest through tailored communications, targeted marketing strategies, and special fan initiatives, ultimately fostering a stronger fan loyalty.
Moreover, the game-day experience has been significantly transformed through data-driven strategies. Analytics allow teams to optimize various aspects of the event, from personalized fan interactions to enhanced stadium amenities. By analyzing fan demographics and purchasing patterns, sports franchises can implement initiatives that ensure a more satisfying experience, such as improving concession offerings based on popular items or enhancing the accessibility of facilities. This focus on data-driven improvements encourages fans to engage more meaningfully with their teams, thereby creating lasting connections that transcend the typical spectator experience.
Furthermore, the role of data analytics in sports broadcasting has grown exponentially. Networks use analytics to delve into audience metrics, which helps them to tailor programming to meet viewer demands. By analyzing viewership data, broadcasters can identify the most appealing content, adjust game coverage strategies, and even influence the timing and nature of advertisements. These dynamic shifts ensure that broadcasts are not only engaging but also relevant to fans’ preferences. As the industry evolves, the continuous integration of analytics into broadcasting serves to create a more immersive viewing experience, bridging the gap between what fans want and what they receive from their sports consumption.
Challenges in Sports Data Analytics
The integration of data analytics within modern sports presents numerous challenges that teams and organizations must navigate. One primary issue is data quality, which encompasses accuracy, consistency, and completeness. Inaccurate data can lead to misguided decisions, adversely affecting team performance and strategy. For example, if a player’s statistics are incorrectly recorded due to unreliable tracking technology, it can misrepresent their abilities and impact recruitment processes. Therefore, ensuring high-quality data is paramount for effective analytics.
Another significant challenge lies in the integration of diverse data sources. Teams often utilize various systems and platforms to collect data, including performance metrics, player health data, and fan engagement statistics. However, aligning these disparate sources into a coherent dataset can be complex and require substantial technical expertise. Moreover, inconsistent formats and terminologies across different sources can hinder effective analysis. Addressing these discrepancies is crucial for gaining holistic insights that accurately reflect team dynamics and performance metrics.
Interpreting sports data is yet another hurdle for teams. The sheer volume of information generated can be overwhelming, leading to difficulties in deriving actionable insights. Coaches and analysts must be proficient in both statistical analysis and the sport itself to translate data into meaningful strategies. This often necessitates extensive training and resources, which can be challenging for smaller organizations with limited budgets. Additionally, there is a human aspect to consider: embracing a data-centric culture within teams. Resistance from players and coaching staff, who may prefer traditional methods, can impede the adoption of analytics. Effective communication and demonstrable results are crucial in fostering acceptance of this evolving approach.
To address these challenges, teams can invest in training programs that elevate data literacy and collaboration among staff. Furthermore, adopting standardized data protocols can streamline integration and improve quality. Through systematic approaches and a committed mindset, teams can enhance their analytics capabilities, ultimately leading to better decision-making and performance outcomes.
The Future of Data Analytics in Sports
The landscape of sports is undergoing significant transformation, primarily driven by advancements in data analytics. As teams and organizations increasingly recognize the value of data in enhancing performance and decision-making processes, emerging trends are shaping the future of sports analytics. Key among these trends are artificial intelligence (AI) and machine learning (ML), which are set to further revolutionize the industry.
AI and ML algorithms offer the possibility of analyzing vast datasets at an unprecedented pace, allowing for real-time insights that were previously unattainable. For instance, these technologies can assess player performance metrics, injury risks, and even predict outcomes based on historical data. This has the potential to shift recruitment strategies, enabling teams to identify talent that may have otherwise flown under the radar. Moreover, predictive analytics can inform coaching tactics and in-game decisions, leading to a more data-driven approach to sports management.
Alongside AI and ML, enhanced data visualization tools are also set to play a crucial role in the sports analytics narrative. These tools enable stakeholders, from coaches to fans, to easily interpret complex data. By presenting information in visually engaging formats, teams can facilitate better communication and understanding among all parties involved. As the accessibility of these tools improves, it is likely that more organizations will embrace data-driven methodologies in their operational frameworks.
However, the integration of advanced analytics raises ethical considerations. The collection and utilization of player data must be approached with caution, ensuring respect for privacy rights and data security. As the industry continues to evolve, establishing clear guidelines and ethical standards will be essential in navigating the multifaceted implications of sports data analytics.
Conclusion
In the dynamic landscape of modern sports, data analytics has emerged as an essential component that transcends mere performance evaluation. This blog post has examined the multifaceted applications of data analytics, ranging from recruitment processes to performance enhancement strategies. The insights gained from data analysis enable teams and organizations to make informed decisions that enhance their competitive edge.
Initially, we explored how data analytics streamlines the recruitment process. By employing sophisticated metrics and analytical techniques, sports teams can identify prospective talent with a higher degree of accuracy. This data-driven approach not only minimizes the risk of recruitment errors but also optimizes resource allocation, allowing organizations to invest wisely in player development.
Furthermore, the role of data analytics in performance optimization was highlighted. Through continuous monitoring and evaluation of players’ statistical performance, coaches and training staff can tailor their strategies to maximize athletes’ strengths while addressing weaknesses. This personalized approach is instrumental in achieving peak performance levels, thereby influencing game outcomes and overall team success.
The landscape of data usage in sports is continuously evolving, driven by advancements in technology and analytical methodologies. Stakeholders, including coaches, team managers, and sports scientists, must adapt to new trends and embrace innovative data-driven strategies to stay competitive. As the accessibility and sophistication of data analytics tools increase, so does the need for a cultural shift towards integrating these analytical techniques into everyday practices within sports organizations.
In summary, the integral role of data analytics in modern sports cannot be overstated. By leveraging the vast amounts of available data, sports entities are positioned to not only enhance their operational effectiveness but also to redefine traditional processes. Embracing data-driven strategies will undoubtedly pave the way for future successes in the sporting world.